💳 The Future of Financial Analysis
AI enhances credit scoring in finance, boosting accuracy and inclusivity with advanced technologies.
Today's Highlights
- How AI is improving credit scoring and risk assessment
- This Week On BuzzBelow - a recap on this week's topics
- In Other News - a few interesting developments we're tracking
Artificial intelligence (AI) has emerged as a transformative force in the rapidly evolving world of finance, particularly in the realms of credit scoring and risk assessment. As traditional methods give way to more advanced technologies, AI is reshaping how financial institutions, lenders, and even individuals approach lending and credit evaluation.
Decision Trees and Random Forests
Decision Trees and Random Forests are used for classifying applicants into different risk categories based on a series of variable decisions. Random forests, an ensemble of decision trees, are particularly effective in reducing overfitting and improving model robustness. IBM offers a range of AI and machine learning products that include credit scoring solutions. Their Watson platform is particularly noted for integrating machine learning to process vast amounts of data.
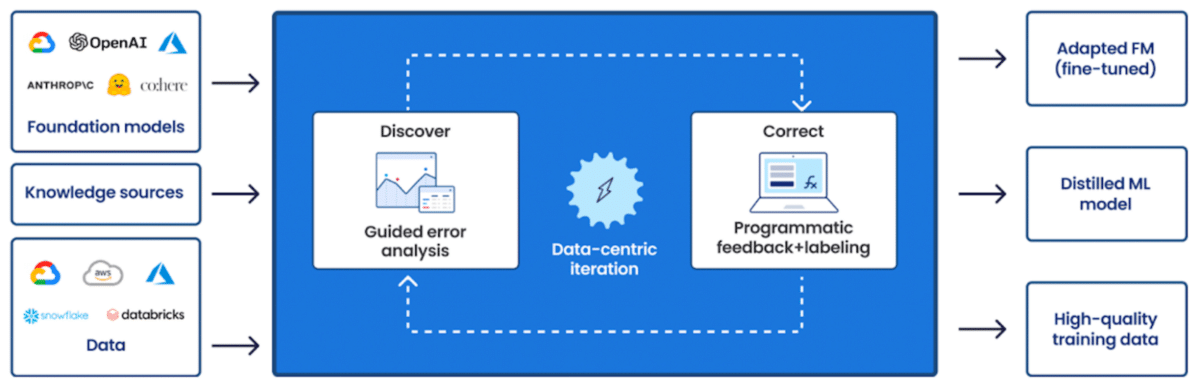
Big Data Analytics
Big data technologies are used to handle and process the volume, variety, and velocity of data needed for AI-driven credit scoring. Technologies such as Hadoop and Spark facilitate the efficient processing of large datasets that are beyond the capability of traditional data processing systems. Cloudera offers software that enables large-scale data processing using platforms like Hadoop and Spark, which are integral to big data analytics in finance.
Implementing AI in Risk Assessment
The implementation of AI in risk assessment involves several stages.
Data collection is gathering data from traditional (e.g., credit history, income) and non-traditional (e.g., smartphone data, browsing patterns) sources.
Data preparation is cleaning and preprocessing data to eliminate errors and inconsistencies which could affect model performance.
Model training is using historical data to train ML models to recognize patterns associated with outcomes such as defaulting on a loan.
Model validation is testing the AI models against a separate set of data to ensure they perform as expected.
Deployment is integrating the AI system into the operational framework of the financial institution for real-time credit scoring and risk assessment.
Continuously monitoring the model's performance and updating it with new data to maintain its accuracy and relevance.
AI models can achieve up to 50% higher accuracy compared to traditional methods. For instance, companies like Upstart and ZestFinance have reported significant improvements in prediction accuracy due to their AI-based models. AI-driven credit scoring and risk assessment are not just passing trends. They represent a significant shift in how financial risk is understood and managed. By leveraging the full spectrum of available data, AI has the power to transform personal finance into a more inclusive, efficient, and consumer-friendly industry.
This Week on BuzzBelow
In Other News
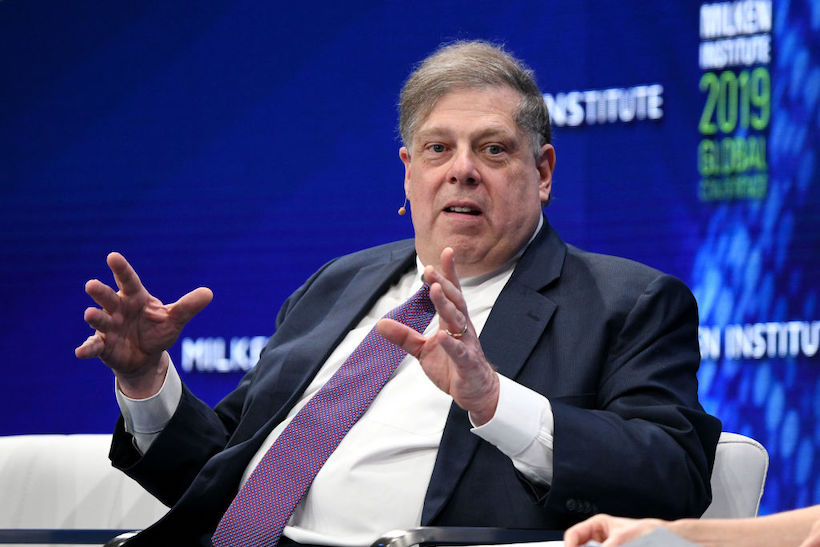
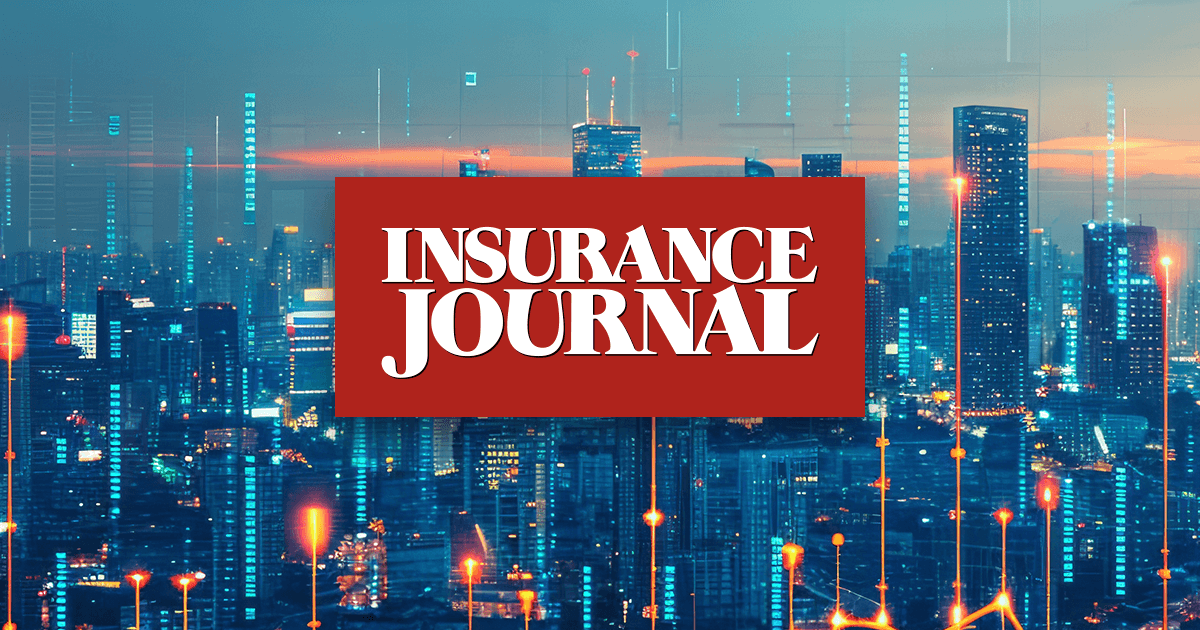
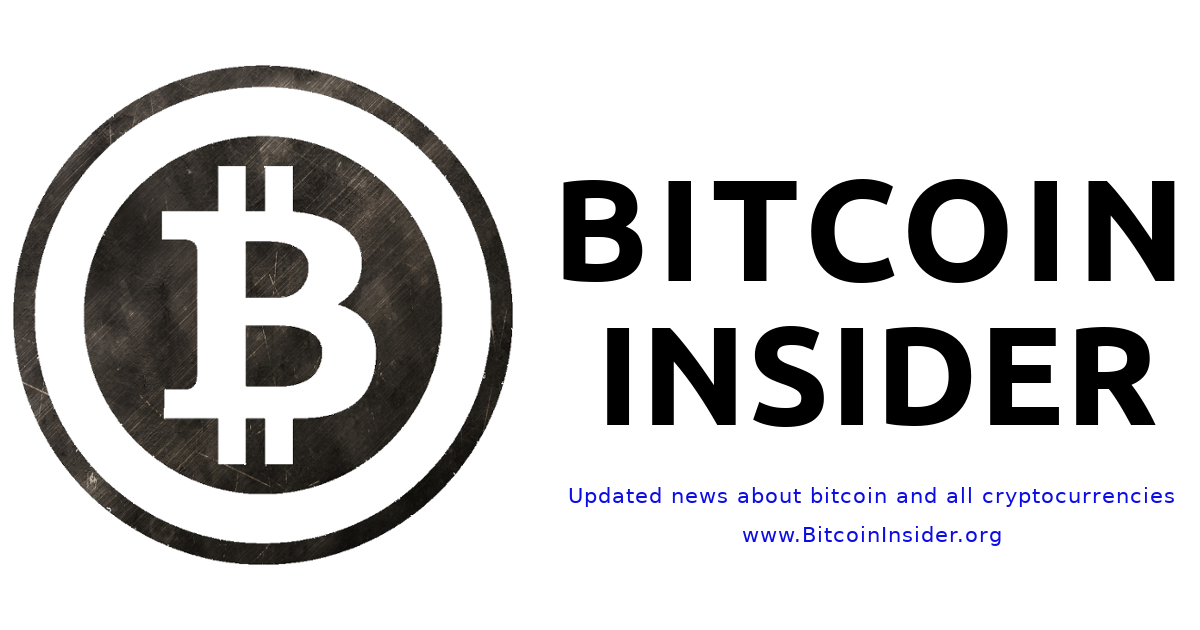