๐พ Bigger Harvests with AI
AI, armed with satellite imagery, sensor data, and weather information, revolutionizes crop yield predictions.
Today's Highlights
- How AI is helping farmers with crop yield prediction
- Learn - a couple of courses to further your knowledge in AI
- AI Jobs - a listing of fresh jobs related to AI
- In Other News - a few interesting developments we're tracking
In recent years, AI has revolutionized agriculture by transforming traditional practices and optimizing crop management. A standout application is AI-driven crop yield prediction, using machine learning, satellite imagery, and IoT devices for unprecedented accuracy. This not only empowers farmers with real-time insights but also fosters sustainable and resource-efficient farming. Spending on AI technologies and solutions alone in Agriculture is predicted to grow from $1 billion in 2020 to $4 billion in 2026, attaining a Compound Annual Growth Rate (CAGR) of 25.5%, according to Markets&Markets.
NASA
NASA's Harvesting the Benefits project uses satellite imagery to monitor and predict crop yields.
- Satellite Imagery: Specialized satellites capture detailed pictures of farmland from space
- Monitoring and Prediction: Images are used to track the health of vegetation, going beyond just observing crops to understanding their well-being
- AI Algorithms: Smart computer algorithms analyze satellite images, trained to recognize patterns and details not easily noticeable to the human eye
- Assessing Vegetation Health: AI algorithms focus on evaluating plant health, identifying signs of robust growth or potential issues like diseases or pests
- Predicting Yield Variations: Based on visual indicators, AI algorithms make predictions about potential variations in crop yields, estimating expected harvest quantities
CLIMATE CORPORATION
Climate Corporation's Climate FieldView platform employs machine learning models to predict crop yields.
- Data Gathering: Collects diverse data, including historical yields, real-time weather, soil details, and field management practices
- Machine Learning Models: Utilizes advanced algorithms to learn from and analyze data patterns, acting like smart computer programs
- Yield Forecasts: Generates accurate predictions by considering various factors and their interactions, providing insights into crop performance
- User-Friendly Interface: Presents forecasted yields through an intuitive interface, enabling farmers to make informed decisions easily for optimal crop management
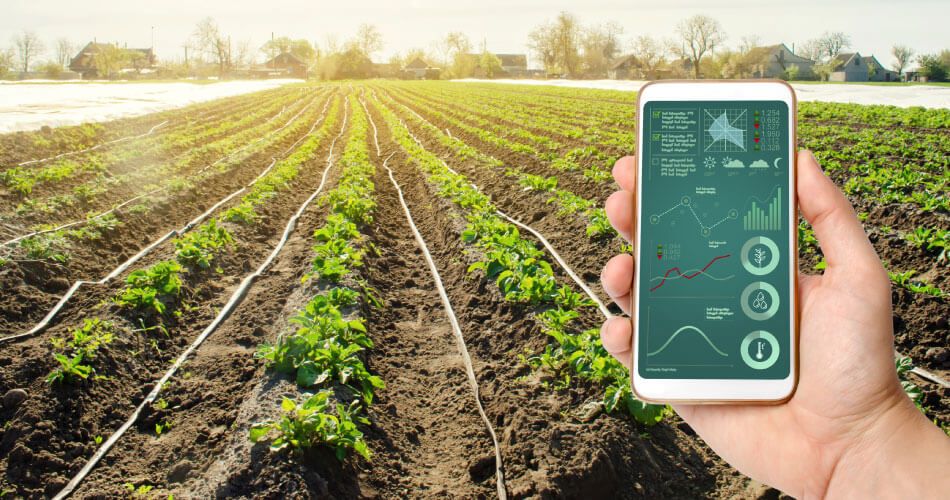
PLANTIX
Plantix, a mobile app, uses AI to identify crop diseases and pests. Farmers can take pictures of affected plants, and the app's AI analyzes the images to diagnose the issue. Early detection allows farmers to take timely action and prevent the spread of diseases, ultimately protecting crop yield.
- User Interaction: Farmers facing crop issues, like diseases or pests, initiate interaction with Plantix for assistance
- Image Capture: Farmers use the app to snap pictures of affected plants using their smartphones, providing visual inputs for AI analysis
- Diagnosis Process: AI algorithms analyze the submitted images in real-time, identifying patterns and characteristics linked to specific diseases or pests
- Diagnostic Results: Plantix swiftly provides farmers with clear and understandable diagnostic results, indicating the likely disease or pest affecting the crop
- User Education: Plantix goes beyond diagnosis, often offering educational features to empower farmers with knowledge for future prevention and effective management
AI in crop yield prediction brings benefits but also faces challenges such as data privacy concerns, potential bias, limited accessibility for small farmers, environmental impact, and the dynamic nature of agriculture. There's also a risk of overreliance on technology, ethical considerations, and algorithm complexity. Addressing these challenges is crucial for responsible and equitable integration in agriculture.
๐ LEARN
Google Cloud
|
University of Illinois at Urbana-Champaign
|
๐งโ๐ป JOBS
Microsoft
|
Mayo Clinic
|
๐ IN OTHER NEWS
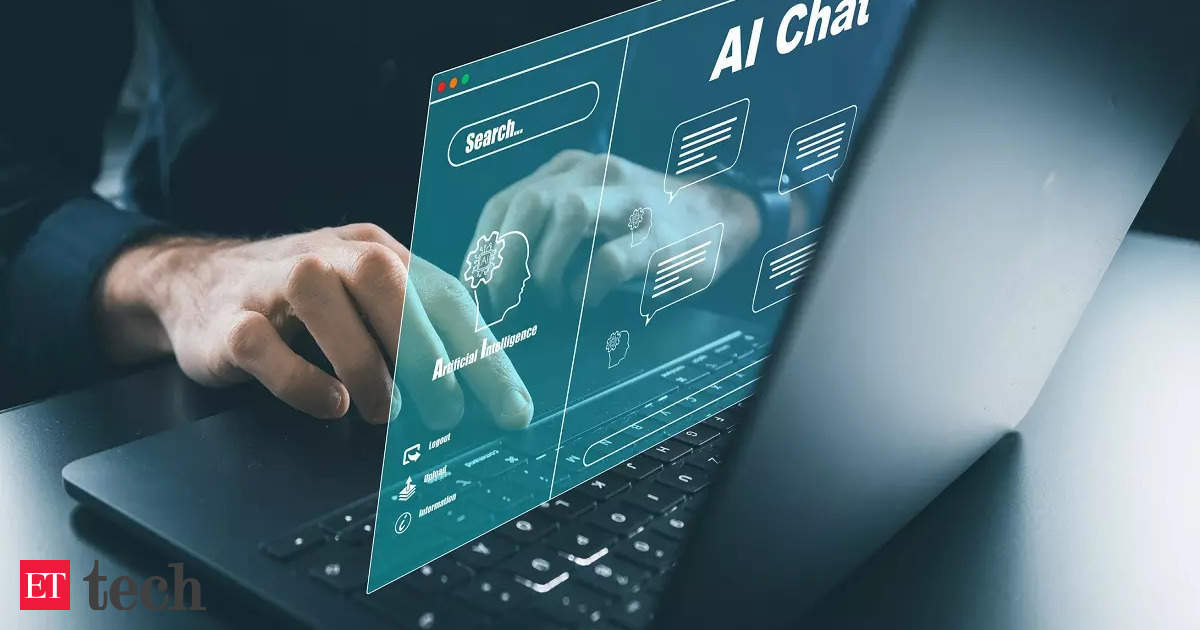
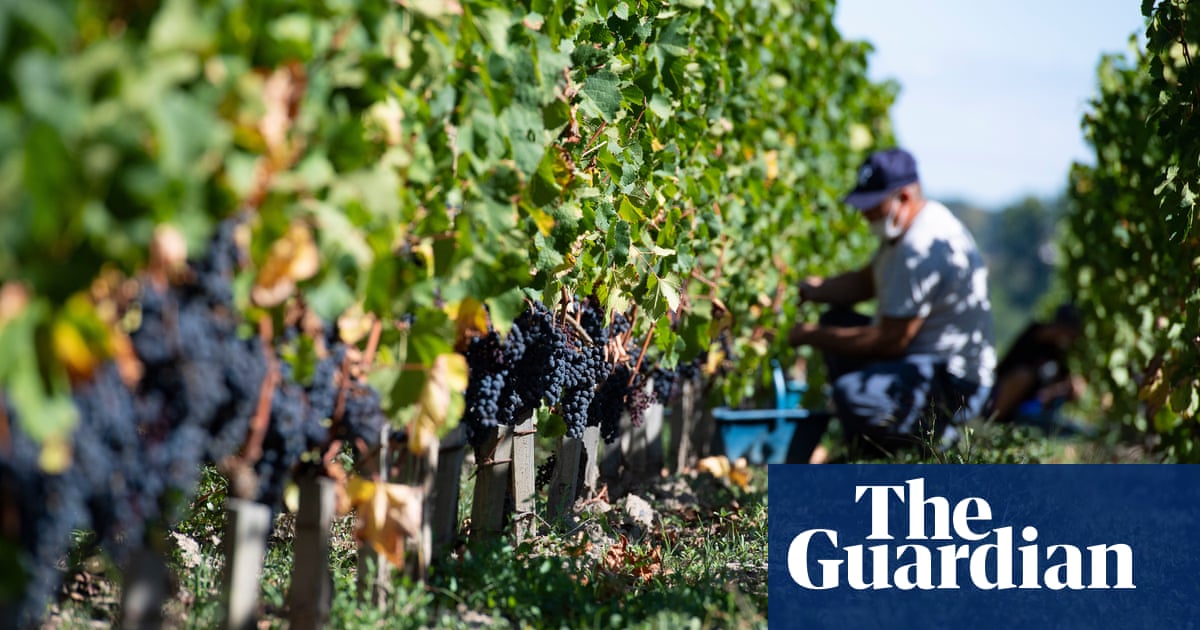
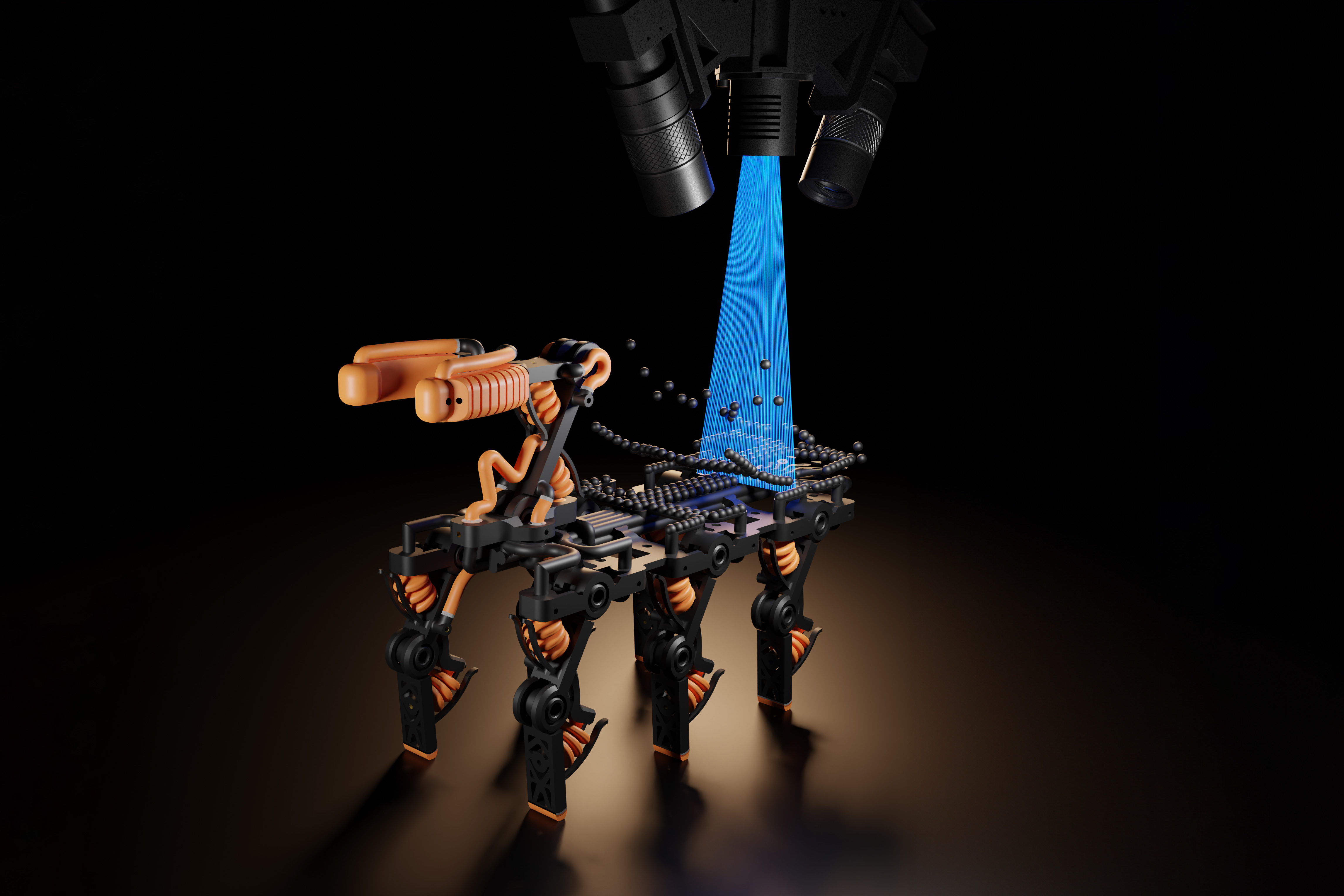