đȘ Astrophysics and AI
AI revolutionizes astrophysics by automating celestial object detection and analysis, thereby enhancing exploration and discovery.
Today's Highlights
- How AI is impacting astrophysics
- This Week On BuzzBelow - a recap on this week's topics
- In Other News - a few interesting developments we're tracking
Artificial Intelligence (AI) has been changing the course of scientific advancements these past few years. Astrophysics has been one of the major fields to be impacted with this technology. In recent years, Artificial Intelligence (AI) has begun to play a crucial role in this domain, particularly in the automated detection and classification of celestial objects.
Automated Feature Extraction and Anomaly Detection
AI algorithms are trained to automatically extract features from astronomical data, which can include characteristics like size, brightness, and spectral properties. Anomaly detection algorithms are particularly important as they can flag unusual or unexpected phenomena in the data, which might signify new discoveries. Techniques like Principal Component Analysis (PCA) and t-Distributed Stochastic Neighbor Embedding (t-SNE) are used to reduce the complexity of data, making it easier to identify key features and patterns. Specializing in Earth observation, Planet Labs employs automated feature extraction algorithms to analyze satellite imagery. This technology is crucial for monitoring changes on Earth but has applications in analyzing other celestial bodies as well.
Synthetic Data Generation and Augmentation
Given the scarcity of labeled astronomical data, synthetic data generation and augmentation techniques are crucial. Generative Adversarial Networks (GANS) and data augmentation methods are used to create additional training data, which enhances the robustness and accuracy of AI models. Utilizing AI for various space exploration projects, Lockheed Martin employs synthetic data generation to simulate space environments and test AI algorithms, ensuring they are robust enough for real-world space applications.
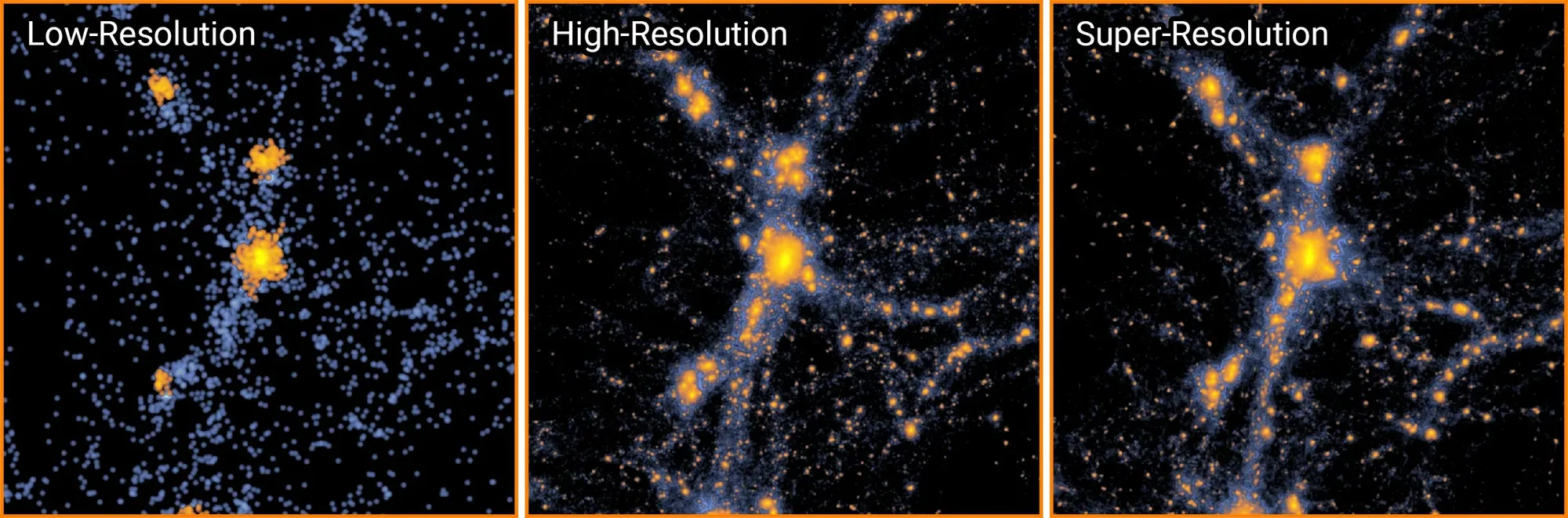
Transfer Learning in Astronomical Surveys
Transfer learning involves applying knowledge gained from one task to another related task. In astrophysics, models trained on one survey can be adapted to analyze data from another survey, even if the characteristics of the data differ. This approach is particularly useful given the unique nature of each astronomical survey. SpaceX's Starlink project, which aims to provide global internet coverage using satellites, also gathers vast amounts of astronomical data. Transfer learning is used here to adapt terrestrial AI models for space-based applications, optimizing satellite operations and data analysis.
With over 20 telescopes having mirrors larger than 20 feet in diameter, the volume of data generated is immense. AI algorithms, particularly neural networks, are essential for processing this data. They have proved to be highly effective in tasks like classifying galaxies, with an accuracy rate of around 98%. AI's role in astrophysics represents a paradigm shift in how we explore and understand the universe. By automating the detection and classification of celestial objects, AI not only enhances our existing knowledge but also opens new frontiers in space exploration. As AI technologies evolve, they promise to unlock deeper mysteries of the cosmos, paving the way for groundbreaking discoveries in astrophysics.
This Week on BuzzBelow
In Other News

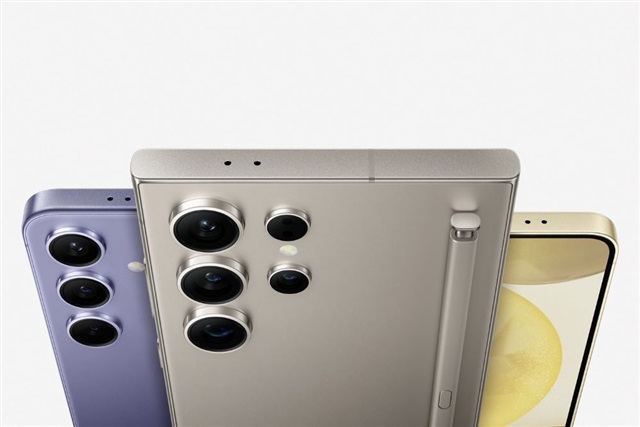
